Derive Asset and Choices/Outcomes Distribution (Analytical)
back to Fan's Dynamic Assets Repository Table of Content.
Contents
- FF_AZ_DS_VECSV finds the stationary asset distributions analytically
- Default
- Parse Parameters
- Start Profiler and Timer
- Get Size of Endogenous and Exogenous State
- 1. Generate Max Index in (NxM) from (N) array.
- 2. Transition Probabilities from (M by M) to (NxM) by M
- 3. Fill mt_pol_idx_mesh_idx to mt_full_trans_mat
- 3.1 Sparse Matrix Approach
- 3.2 Full Matrix Approach
- 4. Stationary Distribution Method A, Eigenvector Approach
- 5. Stationary Distribution Method B, Projection
- 6. Stationary Distribution Method C, Power
- 7. Stationary Vector to Stationary Matrix in Original Dimensions
- End Time and Profiler
- f(y), f(c), f(a): Generate Key Distributional Statistics for Each outcome
function [result_map] = ff_az_ds_vecsv(varargin)
FF_AZ_DS_VECSV finds the stationary asset distributions analytically
Here, we implement the iteration free semi-analytical method for finding asset distributions. The method analytically give the exact stationary distribution induced by the policy function from the dynamic programming problem, conditional on discretizations.
See the appedix of Wang (2019) which develops details on how this works. Suppose endo state size is N and shock is size M, then our transition matrix is (NxM) by (NxM). We know the coh(a,z) value associated with each element of the (NxM) by 1 array. We also know f(a'(a,z),z'|z) transition probability. We contruct a markov chain that has (NxM) states. Specifically:
- We need to transform: mt_pol_idx. This matrix is indexing 1 through N, we need for it to index 1 through (NxM).
- Then we need to duplicate the transition matrix fro shocks.
- Transition Matrix is sparse
Once we have the all states meshed markov transition matrix, then we can use standard methods to find the stationary distribution. Three options are offered here that provide identical solutions:
- The Eigenvector Approach: very fast
- The Projection Approach: medium
- The Power Approach: very slow (especially with sparse matrix)
The program here builds on the Asset Dynamic Programming Problem ff_az_vf_vecsv, here we solve for the asset distribution using vectorized codes. ff_az_ds shows looped codes for finding asset distribution. The solution is the same. Both ff_az_ds and ff_az_ds_vec using optimized-vectorized dynamic programming code from ff_az_vf_vecsv. The idea here is that in addition to vectornizing the dynamic programming funcion, we can also vectorize the distribution code here.
Distributions of Interest:
Statistics include:
- percentiles:
- fraction of outcome held by up to percentiles:
@param param_map container parameter container
@param support_map container support container
@param armt_map container container with states, choices and shocks grids that are inputs for grid based solution algorithm
@param func_map container container with function handles for consumption cash-on-hand etc.
@return result_map container contains policy function matrix, value function matrix, iteration results, and policy function, value function and iteration results tables.
new keys included in result_map in addition to the output from ff_az_vf_vecsv are various distribution statistics for each model outcome, keys include cl_mt_pol_a, cl_mt_pol_c, cl_mt_pol_coh, etc.
@example
% Get Default Parameters it_param_set = 6; [param_map, support_map] = ffs_az_set_default_param(it_param_set); % Change Keys in param_map param_map('it_a_n') = 500; param_map('it_z_n') = 11; param_map('fl_a_max') = 100; param_map('fl_w') = 1.3; % Change Keys support_map support_map('bl_display') = false; support_map('bl_post') = true; support_map('bl_display_final') = false; % Call Program with external parameters that override defaults ff_az_ds_vecsv(param_map, support_map);
@include
@seealso
- derive distribution f(y'(y,z)) one asset loop: ff_az_ds
- derive distribution f(y'({x,y},z)) two assets loop: ff_akz_ds
- derive distribution f(y'({x,y},z, z')) two assets loop: ff_iwkz_ds
- derive distribution f(y'({y},z)) or f(y'({x,y},z)) vectorized: ff_az_ds_vec
- derive distribution f(y'({y},z, z')) or f(y'({x,y},z, z')) vectorized: ff_iwkz_ds_vec
- derive distribution f(y'({y},z)) or f(y'({x,y},z)) semi-analytical: ff_az_ds_vecsv
- derive distribution f(y'({y},z, z')) or f(y'({x,y},z, z')) semi-analytical: ff_iwkz_ds_vecsv
Default
Program can be externally invoked with az, abz or various other programs. By default, program invokes using az model programs:
- it_subset = 5 is basic invoke quick test
- it_subset = 6 is invoke full test
- it_subset = 7 is profiling invoke
- it_subset = 8 is matlab publish
- it_subset = 9 is invoke operational (only final stats) and coh graph
if (~isempty(varargin)) % if invoked from outside override fully [param_map, support_map, armt_map, func_map, result_map] = varargin{:}; else % default invoke close all; it_param_set = 8; bl_input_override = true; % 1. Generate Parameters [param_map, support_map] = ffs_az_set_default_param(it_param_set); % Note: param_map and support_map can be adjusted here or outside to override defaults % param_map('it_a_n') = 750; % param_map('it_z_n') = 15; param_map('st_analytical_stationary_type') = 'eigenvector'; % param_map('st_analytical_stationary_type') = 'projection'; % param_map('st_analytical_stationary_type') = 'power'; % 2. Generate function and grids [armt_map, func_map] = ffs_az_get_funcgrid(param_map, support_map, bl_input_override); % 1 for override % 3. Solve value and policy function using az_vf_vecsv, if want to solve % other models, solve outside then provide result_map as input [result_map] = ff_az_vf_vecsv(param_map, support_map, armt_map, func_map); end
---------------------------------------- ---------------------------------------- xxxxxxxxxxxxxxxxxxxxxxxxxxxxxxxxxxxxxxxx xxxxxxxxxxxxxxxxxxxxxxxxxxxxxxxxxxxxxxxx Begin: Show all key and value pairs from container CONTAINER NAME: SUPPORT_MAP ---------------------------------------- Map with properties: Count: 40 KeyType: char ValueType: any xxxxxxxxxxxxxxxxxxxxxxxxxxxxxxxxxxxxxxxx xxxxxxxxxxxxxxxxxxxxxxxxxxxxxxxxxxxxxxxx ---------------------------------------- ---------------------------------------- pos = 26 ; key = st_img_name_main ; val = ff_az_vf_vecsv_default pos = 27 ; key = st_img_path ; val = C:/Users/fan/CodeDynaAsset//m_az//solve/img/ pos = 28 ; key = st_img_prefix ; val = pos = 29 ; key = st_img_suffix ; val = _p8.png pos = 30 ; key = st_mat_name_main ; val = ff_az_vf_vecsv_default pos = 31 ; key = st_mat_path ; val = C:/Users/fan/CodeDynaAsset//m_az//solve/mat/ pos = 32 ; key = st_mat_prefix ; val = pos = 33 ; key = st_mat_suffix ; val = _p8 pos = 34 ; key = st_mat_test_path ; val = C:/Users/fan/CodeDynaAsset//m_az//test/ff_az_ds_vecsv/mat/ pos = 35 ; key = st_matimg_path_root ; val = C:/Users/fan/CodeDynaAsset//m_az/ pos = 36 ; key = st_profile_name_main ; val = ff_az_vf_vecsv_default pos = 37 ; key = st_profile_path ; val = C:/Users/fan/CodeDynaAsset//m_az//solve/profile/ pos = 38 ; key = st_profile_prefix ; val = pos = 39 ; key = st_profile_suffix ; val = _p8 pos = 40 ; key = st_title_prefix ; val = ---------------------------------------- xxxxxxxxxxxxxxxxxxxxxxxxxxxxxxxxxxxxxxxx Scalars in Container and Sizes and Basic Statistics xxxxxxxxxxxxxxxxxxxxxxxxxxxxxxxxxxxxxxxx i idx value __ ___ _____ bl_display 1 1 0 bl_display_defparam 2 2 1 bl_display_dist 3 3 0 bl_display_final 4 4 0 bl_display_final_dist 5 5 1 bl_display_final_dist_detail 6 6 1 bl_display_funcgrids 7 7 0 bl_graph 8 8 1 bl_graph_coh_t_coh 9 9 1 bl_graph_funcgrids 10 10 0 bl_graph_onebyones 11 11 1 bl_graph_pol_lvl 12 12 0 bl_graph_pol_pct 13 13 0 bl_graph_val 14 14 0 bl_img_save 15 15 0 bl_mat 16 16 0 bl_post 17 17 1 bl_profile 18 18 0 bl_profile_dist 19 19 0 bl_time 20 20 0 it_display_every 21 21 20 it_display_final_colmax 22 22 12 it_display_final_rowmax 23 23 100 it_display_summmat_colmax 24 24 5 it_display_summmat_rowmax 25 25 5 ---------------------------------------- ---------------------------------------- xxxxxxxxxxxxxxxxxxxxxxxxxxxxxxxxxxxxxxxx xxxxxxxxxxxxxxxxxxxxxxxxxxxxxxxxxxxxxxxx Begin: Show all key and value pairs from container CONTAINER NAME: ARMT_MAP ---------------------------------------- Map with properties: Count: 4 KeyType: char ValueType: any xxxxxxxxxxxxxxxxxxxxxxxxxxxxxxxxxxxxxxxx xxxxxxxxxxxxxxxxxxxxxxxxxxxxxxxxxxxxxxxx ---------------------------------------- ---------------------------------------- ---------------------------------------- xxxxxxxxxxxxxxxxxxxxxxxxxxxxxxxxxxxxxxxx Matrix in Container and Sizes and Basic Statistics xxxxxxxxxxxxxxxxxxxxxxxxxxxxxxxxxxxxxxxx i idx rowN colN mean std min max _ ___ ____ ____ ________ ________ _________ _______ ar_a 1 1 1 750 25 14.463 0 50 ar_stationary 2 2 1 15 0.066667 0.060897 0.0027089 0.16757 ar_z 3 3 1 15 1.1347 0.69878 0.34741 2.567 mt_z_trans 4 4 15 15 0.066667 0.095337 0 0.27902 ---------------------------------------- ---------------------------------------- xxxxxxxxxxxxxxxxxxxxxxxxxxxxxxxxxxxxxxxx xxxxxxxxxxxxxxxxxxxxxxxxxxxxxxxxxxxxxxxx Begin: Show all key and value pairs from container CONTAINER NAME: PARAM_MAP ---------------------------------------- Map with properties: Count: 24 KeyType: char ValueType: any xxxxxxxxxxxxxxxxxxxxxxxxxxxxxxxxxxxxxxxx xxxxxxxxxxxxxxxxxxxxxxxxxxxxxxxxxxxxxxxx ---------------------------------------- ---------------------------------------- pos = 23 ; key = st_analytical_stationary_type ; val = eigenvector pos = 24 ; key = st_model ; val = az ---------------------------------------- xxxxxxxxxxxxxxxxxxxxxxxxxxxxxxxxxxxxxxxx Scalars in Container and Sizes and Basic Statistics xxxxxxxxxxxxxxxxxxxxxxxxxxxxxxxxxxxxxxxx i idx value __ ___ _____ bl_loglin 1 1 0 fl_a_max 2 2 50 fl_a_min 3 3 0 fl_b_bd 4 4 0 fl_beta 5 5 0.94 fl_crra 6 6 1.5 fl_loglin_threshold 7 7 1 fl_nan_replace 8 8 -9999 fl_r_save 9 9 0.025 fl_tol_dist 10 10 1e-05 fl_tol_pol 11 11 1e-05 fl_tol_val 12 12 1e-05 fl_w 13 13 1.28 fl_z_mu 14 14 0 fl_z_rho 15 15 0.8 fl_z_sig 16 16 0.2 it_a_n 17 17 750 it_maxiter_dist 18 18 1000 it_maxiter_val 19 19 1000 it_tol_pol_nochange 20 20 25 it_trans_power_dist 21 21 1000 it_z_n 22 22 15 ---------------------------------------- ---------------------------------------- xxxxxxxxxxxxxxxxxxxxxxxxxxxxxxxxxxxxxxxx xxxxxxxxxxxxxxxxxxxxxxxxxxxxxxxxxxxxxxxx Begin: Show all key and value pairs from container CONTAINER NAME: FUNC_MAP ---------------------------------------- Map with properties: Count: 6 KeyType: char ValueType: any xxxxxxxxxxxxxxxxxxxxxxxxxxxxxxxxxxxxxxxx xxxxxxxxxxxxxxxxxxxxxxxxxxxxxxxxxxxxxxxx ---------------------------------------- ---------------------------------------- pos = 1 ; key = f_coh ; val = @(z,b)(z*fl_w+b.*(1+fl_r_save)) pos = 2 ; key = f_cons ; val = @(z,b,bprime)(f_coh(z,b)-bprime) pos = 3 ; key = f_inc ; val = @(z,b)(z*fl_w+b.*(fl_r_save)) pos = 4 ; key = f_util_crra ; val = @(c)(((c).^(1-fl_crra)-1)./(1-fl_crra)) pos = 5 ; key = f_util_log ; val = @(c)log(c) pos = 6 ; key = f_util_standin ; val = @(z,b)f_util_log(f_coh(z,b)) ---------------------------------------- xxxxxxxxxxxxxxxxxxxxxxxxxxxxxxxxxxxxxxxx Scalars in Container and Sizes and Basic Statistics xxxxxxxxxxxxxxxxxxxxxxxxxxxxxxxxxxxxxxxx i idx xFunction _ ___ _________ f_coh 1 1 1 f_cons 2 2 2 f_inc 3 3 3 f_util_crra 4 4 4 f_util_log 5 5 5 f_util_standin 6 6 6 ---------------------------------------- ---------------------------------------- xxxxxxxxxxxxxxxxxxxxxxxxxxxxxxxxxxxxxxxx xxxxxxxxxxxxxxxxxxxxxxxxxxxxxxxxxxxxxxxx Begin: Show all key and value pairs from container CONTAINER NAME: RESULT_MAP ---------------------------------------- Map with properties: Count: 10 KeyType: char ValueType: any xxxxxxxxxxxxxxxxxxxxxxxxxxxxxxxxxxxxxxxx xxxxxxxxxxxxxxxxxxxxxxxxxxxxxxxxxxxxxxxx ---------------------------------------- ---------------------------------------- pos = 2 ; key = ar_st_pol_names ; val = cl_mt_val cl_mt_pol_a cl_mt_coh cl_mt_pol_c ---------------------------------------- xxxxxxxxxxxxxxxxxxxxxxxxxxxxxxxxxxxxxxxx Matrix in Container and Sizes and Basic Statistics xxxxxxxxxxxxxxxxxxxxxxxxxxxxxxxxxxxxxxxx i idx rowN colN mean std min max _ ___ ____ ____ _______ _______ _______ ______ ar_pol_diff_norm 1 1 105 1 29.079 159.48 0 1532.9 ar_val_diff_norm 2 3 105 1 10.915 26.247 0.02899 163.75 cl_mt_coh 3 4 750 15 27.077 14.84 0.44468 54.536 cl_mt_pol_a 4 5 750 15 23.941 13.926 0 49.599 cl_mt_pol_c 5 6 750 15 3.136 0.93512 0.44468 4.9363 cl_mt_val 6 7 750 15 10.288 3.1692 -1.496 15.012 mt_pol_idx 7 8 750 15 359.64 208.62 1 744 mt_pol_perc_change 8 9 105 15 0.21725 0.34614 0 1 mt_val 9 10 750 15 10.288 3.1692 -1.496 15.012
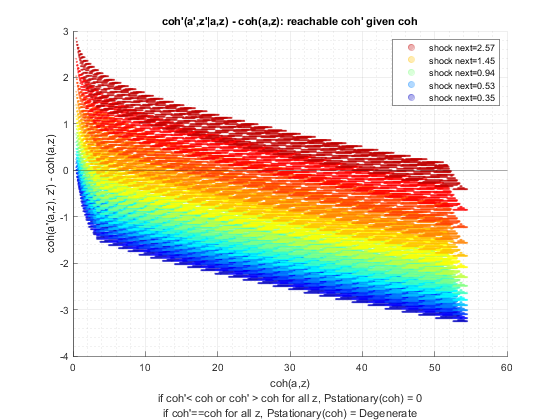
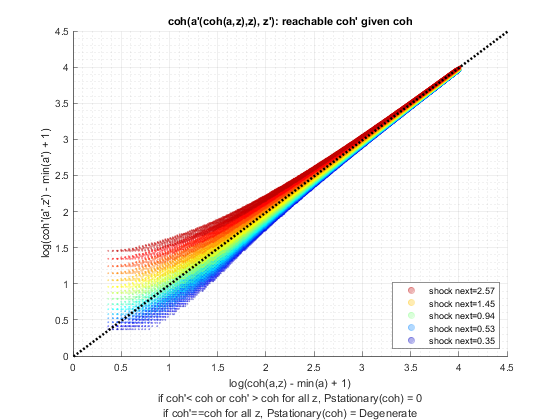
Parse Parameters
% append function name st_func_name = 'ff_az_ds_vecsv'; support_map('st_profile_name_main') = [st_func_name support_map('st_profile_name_main')]; support_map('st_mat_name_main') = [st_func_name support_map('st_mat_name_main')]; support_map('st_img_name_main') = [st_func_name support_map('st_img_name_main')]; % result_map % ar_st_pol_names is from section _Process Optimal Choices_ in the value % function code. params_group = values(result_map, {'mt_pol_idx'}); [mt_pol_idx] = params_group{:}; % armt_map params_group = values(armt_map, {'mt_z_trans'}); [mt_z_trans] = params_group{:}; % param_map params_group = values(param_map, { 'it_trans_power_dist', 'st_analytical_stationary_type'}); [it_trans_power_dist, st_analytical_stationary_type] = params_group{:}; % support_map params_group = values(support_map, {'bl_profile_dist', 'st_profile_path', ... 'st_profile_prefix', 'st_profile_name_main', 'st_profile_suffix',... 'bl_time'}); [bl_profile_dist, st_profile_path, ... st_profile_prefix, st_profile_name_main, st_profile_suffix, ... bl_time] = params_group{:};
Start Profiler and Timer
% Start Profile if (bl_profile_dist) close all; profile off; profile on; end % Start Timer if (bl_time) tic; end
Get Size of Endogenous and Exogenous State
The key idea is that all information for policy function is captured by mt_pol_idx matrix, its rows are the number of endogenous states, and its columns are the exogenous shocks.
[it_endostates_rows_n, it_exostates_cols_n] = size(mt_pol_idx);
1. Generate Max Index in (NxM) from (N) array.
Suppose we have:
mt_pol_idx =
1 1 2 2 2 2 3 3 3 4 4 4 4 5 5
These become:
mt_pol_idx_mesh_max =
1 6 11 2 7 12 3 8 13 4 9 14 5 10 15 1 6 11 2 7 12 3 8 13 4 9 14 5 10 15 1 6 11 2 7 12 3 8 13 4 9 14 5 10 15
% mt_pol_idx_mesh_max is (NxM) by M, mt_pol_idx is N by M
mt_pol_idx_mesh_max = mt_pol_idx(:) + (0:1:(it_exostates_cols_n-1))*it_endostates_rows_n;
2. Transition Probabilities from (M by M) to (NxM) by M
mt_trans_prob =
0.9332 0.0668 0.0000 0.9332 0.0668 0.0000 0.9332 0.0668 0.0000 0.9332 0.0668 0.0000 0.9332 0.0668 0.0000 0.0062 0.9876 0.0062 0.0062 0.9876 0.0062 0.0062 0.9876 0.0062 0.0062 0.9876 0.0062 0.0062 0.9876 0.0062 0.0000 0.0668 0.9332 0.0000 0.0668 0.9332 0.0000 0.0668 0.9332 0.0000 0.0668 0.9332 0.0000 0.0668 0.9332
mt_trans_prob = reshape(repmat(mt_z_trans(:)', [it_endostates_rows_n, 1]), [it_endostates_rows_n*it_exostates_cols_n, it_exostates_cols_n]);
3. Fill mt_pol_idx_mesh_idx to mt_full_trans_mat
Try to always use sparse matrix, unless grid sizes very small, keeping non-sparse code here for comparison. Sparse matrix is important for allowing the code to be fast and memory efficient. Otherwise this method is much slower than iterative method.
it_sparse_threshold = 100*7;
if (it_endostates_rows_n*it_exostates_cols_n > it_sparse_threshold)
3.1 Sparse Matrix Approach
i = mt_pol_idx_mesh_max(:); j = repmat((1:1:it_endostates_rows_n*it_exostates_cols_n),[1,it_exostates_cols_n])'; v = mt_trans_prob(:); m = it_endostates_rows_n*it_exostates_cols_n; n = it_endostates_rows_n*it_exostates_cols_n; mt_full_trans_mat = sparse(i, j, v, m, n);
else
3.2 Full Matrix Approach
ar_lin_idx_start_point =
0 15 30 45 60 75 90 105 120 135 150 165 180 195 210
mt_pol_idx_mesh_idx_meshfull =
1 6 11 17 22 27 33 38 43 49 54 59 65 70 75 76 81 86 92 97 102 108 113 118 124 129 134 140 145 150 151 156 161 167 172 177 183 188 193 199 204 209 215 220 225
% Each row's linear index starting point ar_lin_idx_start_point = ((it_endostates_rows_n*it_exostates_cols_n)*(0:1:(it_endostates_rows_n*it_exostates_cols_n-1))); % mt_pol_idx_mesh_idx_meshfull is (NxM) by M % Full index in (NxM) to (NxM) transition Matrix mt_pol_idx_mesh_idx_meshfull = mt_pol_idx_mesh_max + ar_lin_idx_start_point'; % Fill mt_pol_idx_mesh_idx to mt_full_trans_mat mt_full_trans_mat = zeros([it_endostates_rows_n*it_exostates_cols_n, it_endostates_rows_n*it_exostates_cols_n]); mt_full_trans_mat(mt_pol_idx_mesh_idx_meshfull(:)) = mt_trans_prob(:);
end
4. Stationary Distribution Method A, Eigenvector Approach
Given that markov chain we have constructured for all state-space elements, we can now find the stationary distribution using standard eigenvector approach.
if (strcmp(st_analytical_stationary_type, 'eigenvector')) [V, ~] = eigs(mt_full_trans_mat,1,1); ar_stationary = V/sum(V); end
5. Stationary Distribution Method B, Projection
This uses Projection.
if (strcmp(st_analytical_stationary_type, 'projection')) % a. Transition - I % P = P*T % 0 = P*T - P % 0 = P*(T-1) % Q = trans_prob - np.identity(state_count); mt_diag = eye(it_endostates_rows_n*it_exostates_cols_n); if (it_endostates_rows_n*it_exostates_cols_n > it_sparse_threshold) % if larger, use sparse matrix mt_diag = sparse(mt_diag); end mt_Q = mt_full_trans_mat' - mt_diag; % b. add all 1 as final column, (because P*1 = 1) % one_col = np.ones((state_count,1)) % Q = np.column_stack((Q, one_col)) ar_one = ones([it_endostates_rows_n*it_exostates_cols_n,1]); mt_Q = [mt_Q, ar_one]; % c. b is the LHS % b = [0,0,0,...,1] % b = np.zeros((1, (state_count+1))) % b[0, state_count] = 1 ar_b = zeros([1, it_endostates_rows_n*it_exostates_cols_n+1]); if (it_endostates_rows_n*it_exostates_cols_n > it_sparse_threshold) % if larger, use sparse matrix ar_b = sparse(ar_b); end ar_b(it_endostates_rows_n*it_exostates_cols_n+1) = 1; % d. solve % b = P*Q % b*Q^{T} = P*Q*Q^{T} % P*Q*Q^{T} = b*Q^{T} % P = (b*Q^{T})[(Q*Q^{T})^{-1}] % Q_t = np.transpose(Q) % b_QT = np.dot(b, Q_t) % Q_QT = np.dot(Q, Q_t) % inv_mt_Q_QT = inv(mt_Q*mt_Q'); ar_stationary = (ar_b*mt_Q')/(mt_Q*mt_Q'); end
6. Stationary Distribution Method C, Power
Takes markov chain to Nth power. This is the slowest.
if (strcmp(st_analytical_stationary_type, 'power')) mt_stationary_full = (mt_full_trans_mat)^it_trans_power_dist; ar_stationary = mt_stationary_full(:,1); end
7. Stationary Vector to Stationary Matrix in Original Dimensions
mt_dist_az = reshape(ar_stationary, size(mt_pol_idx));
End Time and Profiler
% End Timer if (bl_time) toc; end % End Profile if (bl_profile_dist) profile off profile viewer st_file_name = [st_profile_prefix st_profile_name_main st_profile_suffix]; profsave(profile('info'), strcat(st_profile_path, st_file_name)); end
f(y), f(c), f(a): Generate Key Distributional Statistics for Each outcome
Having derived f(a,z) the probability mass function of the joint discrete random variables, we now obtain distributional statistics. Note that we know f(a,z), and we also know relevant policy functions a'(a,z), c(a,z), or other policy functions. We can simulate any choices that are a function of the random variables (a,z), using f(a,z). We call function ff_az_ds_post_stats which uses fft_disc_rand_var_stats and fft_disc_rand_var_mass2outcomes to compute various statistics of interest.
bl_input_override = true;
result_map('mt_dist') = mt_dist_az;
result_map = ff_az_ds_post_stats(support_map, result_map, mt_dist_az, bl_input_override);
---------------------------------------- xxxxxxxxxxxxxxxxxxxxxxxxxxxxxxxxxxxxxxxx Summary Statistics for: cl_mt_val xxxxxxxxxxxxxxxxxxxxxxxxxxxxxxxxxxxxxxxx ---------------------------------------- fl_choice_mean 3.2157 fl_choice_sd 1.5948 fl_choice_coefofvar 0.4959 fl_choice_prob_zero 0 fl_choice_prob_below_zero 0.0237 fl_choice_prob_above_zero 0.9763 fl_choice_prob_max 7.6404e-37 tb_disc_cumu cl_mt_valDiscreteVal cl_mt_valDiscreteValProbMass CDF cumsumFrac ____________________ ____________________________ _______ __________ -1.496 0.0022504 0.22504 -0.0010469 -1.2889 0.00011697 0.23674 -0.0010938 -1.1195 5.0108e-05 0.24175 -0.0011113 -0.96773 5.0841e-05 0.24683 -0.0011266 -0.85738 0.0054609 0.79292 -0.0025826 -0.82642 2.8066e-05 0.79573 -0.0025898 -0.69878 2.9571e-05 0.79869 -0.0025962 -0.68792 0.00036428 0.83512 -0.0026742 -0.57856 2.2451e-05 0.83736 -0.0026782 -0.54598 0.000144 0.85176 -0.0027026 cl_mt_valDiscreteVal cl_mt_valDiscreteValProbMass CDF cumsumFrac ____________________ ____________________________ ___ __________ 14.956 -4.1119e-38 100 1 14.962 -5.9703e-37 100 1 14.968 -3.0565e-37 100 1 14.975 -8.9711e-37 100 1 14.981 -1.4634e-36 100 1 14.987 3.3654e-39 100 1 14.993 3.5858e-36 100 1 14.999 -4.6852e-38 100 1 15.006 9.0524e-37 100 1 15.012 7.6404e-37 100 1 tb_prob_drv percentiles cl_mt_valDiscreteValPercentileValues fracOfSumHeldBelowThisPercentile ___________ ____________________________________ ________________________________ 0.1 -1.496 -0.0010469 1 -0.20677 -0.0036092 5 0.4326 0.00013299 10 1.051 0.016603 15 1.6461 0.054888 20 1.7912 0.064607 25 2.2186 0.1208 35 2.6167 0.16828 50 3.239 0.30279 65 3.834 0.46984 75 4.2838 0.59112 80 4.5488 0.66006 85 4.8767 0.73292 90 5.2801 0.81147 95 5.8899 0.89785 99 7.0098 0.97685 99.9 8.0879 0.99741 ---------------------------------------- xxxxxxxxxxxxxxxxxxxxxxxxxxxxxxxxxxxxxxxx Summary Statistics for: cl_mt_pol_a xxxxxxxxxxxxxxxxxxxxxxxxxxxxxxxxxxxxxxxx ---------------------------------------- fl_choice_mean 0.8302 fl_choice_sd 1.1777 fl_choice_coefofvar 1.4186 fl_choice_prob_zero 0.2816 fl_choice_prob_below_zero 0 fl_choice_prob_above_zero 0.7184 fl_choice_prob_max 7.6404e-37 tb_disc_cumu cl_mt_pol_aDiscreteVal cl_mt_pol_aDiscreteValProbMass CDF cumsumFrac ______________________ ______________________________ ______ __________ 0 0.2816 28.16 0 0.066756 0.06379 34.539 0.0051292 0.13351 0.035757 38.115 0.01088 0.20027 0.051963 43.311 0.023414 0.26702 0.034354 46.746 0.034464 0.33378 0.034466 50.193 0.04832 0.40053 0.033489 53.542 0.064477 0.46729 0.023335 55.875 0.077611 0.53405 0.030341 58.91 0.097129 0.6008 0.023923 61.302 0.11444 cl_mt_pol_aDiscreteVal cl_mt_pol_aDiscreteValProbMass CDF cumsumFrac ______________________ ______________________________ ___ __________ 48.999 3.7544e-37 100 1 49.065 -2.3412e-36 100 1 49.132 4.1418e-37 100 1 49.199 -5.653e-37 100 1 49.266 -1.4634e-36 100 1 49.332 3.3654e-39 100 1 49.399 3.5858e-36 100 1 49.466 -4.6852e-38 100 1 49.533 9.0524e-37 100 1 49.599 7.6404e-37 100 1 tb_prob_drv percentiles cl_mt_pol_aDiscreteValPercentileValues fracOfSumHeldBelowThisPercentile ___________ ______________________________________ ________________________________ 0.1 0 0 1 0 0 5 0 0 10 0 0 15 0 0 20 0 0 25 0 0 35 0.13351 0.01088 50 0.33378 0.04832 65 0.73431 0.14695 75 1.1348 0.26012 80 1.4686 0.35487 85 1.8024 0.43805 90 2.3364 0.56666 95 3.271 0.73106 99 5.3405 0.92164 99.9 8.0774 0.98952 ---------------------------------------- xxxxxxxxxxxxxxxxxxxxxxxxxxxxxxxxxxxxxxxx Summary Statistics for: cl_mt_coh xxxxxxxxxxxxxxxxxxxxxxxxxxxxxxxxxxxxxxxx ---------------------------------------- fl_choice_mean 2.1310 fl_choice_sd 1.4656 fl_choice_coefofvar 0.6878 fl_choice_prob_zero 0 fl_choice_prob_below_zero 0 fl_choice_prob_above_zero 1.0000 fl_choice_prob_max 7.6404e-37 tb_disc_cumu cl_mt_cohDiscreteVal cl_mt_cohDiscreteValProbMass CDF cumsumFrac ____________________ ____________________________ _______ __________ 0.44468 0.0022504 0.22504 0.00046961 0.51297 0.0054609 0.77113 0.0017842 0.51311 0.00011697 0.78283 0.0018123 0.5814 0.00036428 0.81926 0.0019117 0.58153 5.0108e-05 0.82427 0.0019254 0.59175 0.013554 2.1797 0.0056892 0.64982 0.000144 2.1941 0.0057331 0.64996 5.0841e-05 2.1991 0.0057486 0.66017 0.0011392 2.3131 0.0061015 0.68262 0.027201 5.0332 0.014815 cl_mt_cohDiscreteVal cl_mt_cohDiscreteValProbMass CDF cumsumFrac ____________________ ____________________________ ___ __________ 54.03 7.1983e-37 100 1 54.057 -3.0565e-37 100 1 54.098 3.318e-37 100 1 54.125 -8.9711e-37 100 1 54.194 -1.4634e-36 100 1 54.262 3.3654e-39 100 1 54.331 3.5858e-36 100 1 54.399 -4.6852e-38 100 1 54.467 9.0524e-37 100 1 54.536 7.6404e-37 100 1 tb_prob_drv percentiles cl_mt_cohDiscreteValPercentileValues fracOfSumHeldBelowThisPercentile ___________ ____________________________________ ________________________________ 0.1 0.44468 0.00046961 1 0.59175 0.0056892 5 0.68262 0.014815 10 0.85587 0.035385 15 0.90837 0.06043 20 1.0479 0.098946 25 1.1136 0.10384 35 1.2772 0.15923 50 1.6681 0.2648 65 2.1977 0.39995 75 2.6879 0.51075 80 3.0188 0.57803 85 3.4427 0.65282 90 4.0585 0.74062 95 5.1064 0.84656 99 7.4642 0.95922 99.9 10.402 0.99464 ---------------------------------------- xxxxxxxxxxxxxxxxxxxxxxxxxxxxxxxxxxxxxxxx Summary Statistics for: cl_mt_pol_c xxxxxxxxxxxxxxxxxxxxxxxxxxxxxxxxxxxxxxxx ---------------------------------------- fl_choice_mean 1.3008 fl_choice_sd 0.3450 fl_choice_coefofvar 0.2652 fl_choice_prob_zero 0 fl_choice_prob_below_zero 0 fl_choice_prob_above_zero 1.0000 fl_choice_prob_max 7.6404e-37 tb_disc_cumu cl_mt_pol_cDiscreteVal cl_mt_pol_cDiscreteValProbMass CDF cumsumFrac ______________________ ______________________________ _______ __________ 0.44468 0.0022504 0.22504 0.00076933 0.51297 0.0054609 0.77113 0.0029229 0.51311 0.00011697 0.78283 0.0029691 0.5814 0.00036428 0.81926 0.0031319 0.58153 5.0108e-05 0.82427 0.0031543 0.5832 5.0841e-05 0.82935 0.0031771 0.59175 0.013554 2.1847 0.0093431 0.64982 0.000144 2.1991 0.0094151 0.65149 0.00017056 2.2162 0.0095005 0.65163 2.8066e-05 2.219 0.0095145 cl_mt_pol_cDiscreteVal cl_mt_pol_cDiscreteValProbMass CDF cumsumFrac ______________________ ______________________________ ___ __________ 4.9213 -4.1119e-38 100 1 4.923 -5.9703e-37 100 1 4.9246 -3.0565e-37 100 1 4.9263 -8.9711e-37 100 1 4.928 -1.4634e-36 100 1 4.9297 3.3654e-39 100 1 4.9313 3.5858e-36 100 1 4.933 -4.6852e-38 100 1 4.9347 9.0524e-37 100 1 4.9363 7.6404e-37 100 1 tb_prob_drv percentiles cl_mt_pol_cDiscreteValPercentileValues fracOfSumHeldBelowThisPercentile ___________ ______________________________________ ________________________________ 0.1 0.44468 0.00076933 1 0.59175 0.0093431 5 0.68262 0.024382 10 0.81947 0.054893 15 0.90837 0.10337 20 1.0257 0.12476 25 1.0479 0.17681 35 1.1964 0.25339 50 1.3276 0.41348 65 1.4161 0.55331 75 1.5268 0.6673 80 1.5952 0.72762 85 1.6619 0.78838 90 1.7521 0.85358 95 1.8668 0.92271 99 2.1241 0.98298 99.9 2.3562 0.99811 OriginalVariableNames cl_mt_val cl_mt_pol_a cl_mt_coh cl_mt_pol_c _____________________ __________ ___________ __________ ___________ 'mean' 3.2157 0.83022 2.131 1.3008 'sd' 1.5948 1.1777 1.4656 0.34502 'coefofvar' 0.49595 1.4186 0.68776 0.26525 'min' -1.496 0 0.44468 0.44468 'max' 15.012 49.599 54.536 4.9363 'pYis0' 0 0.2816 0 0 'pYls0' 0.023708 0 0 0 'pYgr0' 0.97629 0.7184 1 1 'pYisMINY' 0.0022504 0.2816 0.0022504 0.0022504 'pYisMAXY' 7.6404e-37 7.6404e-37 7.6404e-37 7.6404e-37 'p0_1' -1.496 0 0.44468 0.44468 'p1' -0.20677 0 0.59175 0.59175 'p5' 0.4326 0 0.68262 0.68262 'p10' 1.051 0 0.85587 0.81947 'p15' 1.6461 0 0.90837 0.90837 'p20' 1.7912 0 1.0479 1.0257 'p25' 2.2186 0 1.1136 1.0479 'p35' 2.6167 0.13351 1.2772 1.1964 'p50' 3.239 0.33378 1.6681 1.3276 'p65' 3.834 0.73431 2.1977 1.4161 'p75' 4.2838 1.1348 2.6879 1.5268 'p80' 4.5488 1.4686 3.0188 1.5952 'p85' 4.8767 1.8024 3.4427 1.6619 'p90' 5.2801 2.3364 4.0585 1.7521 'p95' 5.8899 3.271 5.1064 1.8668 'p99' 7.0098 5.3405 7.4642 2.1241 'p99_9' 8.0879 8.0774 10.402 2.3562 'fl_cov_cl_mt_val' 2.5434 1.464 2.0116 0.54759 'fl_cor_cl_mt_val' 1 0.77944 0.86061 0.99518 'fl_cov_cl_mt_pol_a' 1.464 1.387 1.708 0.32097 'fl_cor_cl_mt_pol_a' 0.77944 1 0.98953 0.78991 'fl_cov_cl_mt_coh' 2.0116 1.708 2.148 0.44001 'fl_cor_cl_mt_coh' 0.86061 0.98953 1 0.87016 'fl_cov_cl_mt_pol_c' 0.54759 0.32097 0.44001 0.11904 'fl_cor_cl_mt_pol_c' 0.99518 0.78991 0.87016 1 'fracByP0_1' -0.0010469 0 0.00046961 0.00076933 'fracByP1' -0.0036092 0 0.0056892 0.0093431 'fracByP5' 0.00013299 0 0.014815 0.024382 'fracByP10' 0.016603 0 0.035385 0.054893 'fracByP15' 0.054888 0 0.06043 0.10337 'fracByP20' 0.064607 0 0.098946 0.12476 'fracByP25' 0.1208 0 0.10384 0.17681 'fracByP35' 0.16828 0.01088 0.15923 0.25339 'fracByP50' 0.30279 0.04832 0.2648 0.41348 'fracByP65' 0.46984 0.14695 0.39995 0.55331 'fracByP75' 0.59112 0.26012 0.51075 0.6673 'fracByP80' 0.66006 0.35487 0.57803 0.72762 'fracByP85' 0.73292 0.43805 0.65282 0.78838 'fracByP90' 0.81147 0.56666 0.74062 0.85358 'fracByP95' 0.89785 0.73106 0.84656 0.92271 'fracByP99' 0.97685 0.92164 0.95922 0.98298 'fracByP99_9' 0.99741 0.98952 0.99464 0.99811
end
ans = Map with properties: Count: 13 KeyType: char ValueType: any